Endo SIG Tech Update: Artificial Intelligence
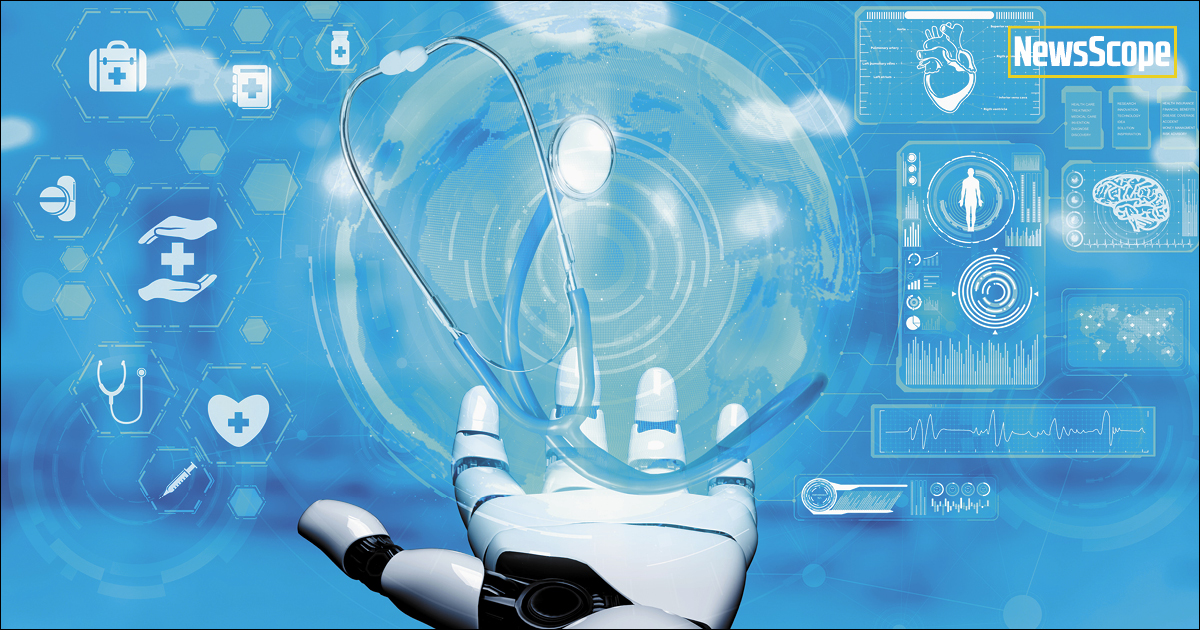
Artificial intelligence (AI) is a broad term given to computer algorithms that are able to execute at the same level or improved at activities traditionally learned by the human brain. AI in medicine refers to the application of such technology in the diagnosis and treatment of disease. Such technology can also be beneficial for the gynecologist.
Machine learning, a subfield of artificial intelligence, is the ability to learn from the data without explicitly being programmed by extracting patterns from data.
Deep learning, a component/subset of machine learning, relies on a large amount of data for training multiple layers of connections similar to the human brain called neural networks (NN) or artificial neural networks (ANN). ANNs are great at extracting patterns/features that are invisible to naked eye and hidden in the medical image data. ANNs have an input layer , multiple hidden( deep ) layers and an output layer. Deep learning can be done supervised, where pathology is labeled which is human labor-intensive or unsupervised in which the ANN will understand the differences between the normal and abnormal images and cluster them together. Imaging and large data digital base of medical imaging data such MRI , CT , US are a great opportunity for advancing AI. Ultrasound has its unique challenges related to variability in the collected data (manual data collection, variability in ultrasound machine acquisition settings) and the clinician interpretation1. Deep learning is favorable for ultrasound applications as the predictable acoustic patterns are not commonly observable.
Typical AI in medicine applications involves classification, segmentation, prediction, and registration. There is ongoing research in multiple disciplines such as breast, prostate, heart, kidney spleen, lung thyroid. There are only 11FDA cleared AI algorithms, none in obstetrics and gynecology2. In obstetrics and gynecology, the research focus has been on the placenta, fetal biometry, fetal scan completeness and anomaly identification, preterm birth. In gynecology, early applications were related to the identification of ovarian masses and probability of malignancy and urinary incontinence3. Since 2017, there is a limited resurgence in the research and publications related to endometrium evaluation. The endometrium has been evaluated with CNN segmentation with potential clinical use for early endometrial cancer detection and endometrial polyps. Regarding endometriosis, only one publication mentioned preoperative obliteration of the cul de sac prediction through sliding sign evaluation was similar between CNN approach and experienced gynecologic sonographic evaluation4.
There is research in progress looking endometriosis diagnosis and ultrasound using multifeature data such as B-mode US data, radio frequency (RF) US signal data, and conventional screening tests5. The objective is to design a deep learning framework for accurate and robust early diagnosis of endometriosis by leveraging multi feature information. Ultrasound has an advantage of be able to obtain a live video and 3 D images which has more digital information and likely will play in the future. Intraoperative use of AI and ultrasound for endometriosis detection could be another developing direction as suggested by the oncological work.
This field is rapidly advancing and health care providers needs to be active participants in defining the clinical applications. A team approach between the AI scientists and clinicians will likely provide the best patient outcomes. As the gold standard for endometriosis diagnosis is laparoscopy , the gynecologists will have an important role in validating these algorithms as diagnostic tools.
References
1 L. Drukker, J. A. Noble ,A. T. Papageorghiou “Introduction to artificial intelligence in ultrasound imaging in obstetrics and gynecology” Ultrasound Obstet Gynecol .First published: 12 June 2020 https://doi.org/10.1002/uog.22122
2. https://www.acrdsi.org/dsi-services/fda-cleared-ai-algorithms
3. F. Christiansen E. L. Epstein E. Smedberg M. Åkerlund K. Smith E. Epstein
“Ultrasound image analysis using deep neural networks for discriminating between benign and malignant ovarian tumors: comparison with expert subjective assessment” Ultrasound Obstet Gynecol 2021; 57: 155–163 DOI: 10.1002/uog.23530.
4. K. O’Shea “Deep neural networks for predicting pouch of Douglas obliteration based on transvaginal ultrasound sliding sign videos,” Ultrasound in Obstetrics & Gynecology 2016; 48 (Suppl. 1): 270–393
5. I. Hacihaliloglu, A. Balica “A Method of Improving Representation of Endometriosis Ultrasound Data By Using Local Phase Tissue Signature”s Journal of Minimally Invasive GynecologyVol. 27Issue 7S112Published in issue: November, 2020